Import Images from Tarfile into SQLite
After processing all my image tarfiles and save a thumbnail tarfile per folder it is time to save all the thumbnails into an SQLite database.
The great sqlite-utils library from Simon Willison has already an insert-files
command, but this needs files on the disk (or stdin magic).
Me reading images from a tarfile is probably too niche to add as a feature, so the code is not using the CLI part of sqlite-utils, but the Python API.
Reading images from tarfile and write them into SQLite in Python:
import datetime import tarfile from pathlib import Path import click from sqlite_utils.db import Database @click.command() @click.option("-f", "--thumbfile") def main(thumbfile): db = Database("gopro_images.db") table = db["thumbnails"] thumb_fn = Path(thumbfile) folder_name = thumb_fn.parent.name with tarfile.open(thumb_fn, "r") as tar_thumbs: for member in tar_thumbs: name = member.name.split("/")[-1] fp = tar_thumbs.extractfile(member) contents = fp.read() table.upsert({ "id": f"{folder_name}/{name}", "content": contents, "size": len(contents), }, pk="id") if __name__ == "__main__": main()
The "id" field is the foldername and the name of the image from the tarfile.
Thanks to the datasette-render-image plugin the images (stored as binary content) can be rendered within datasette. Example screenshot with a some metadata (date and bike):
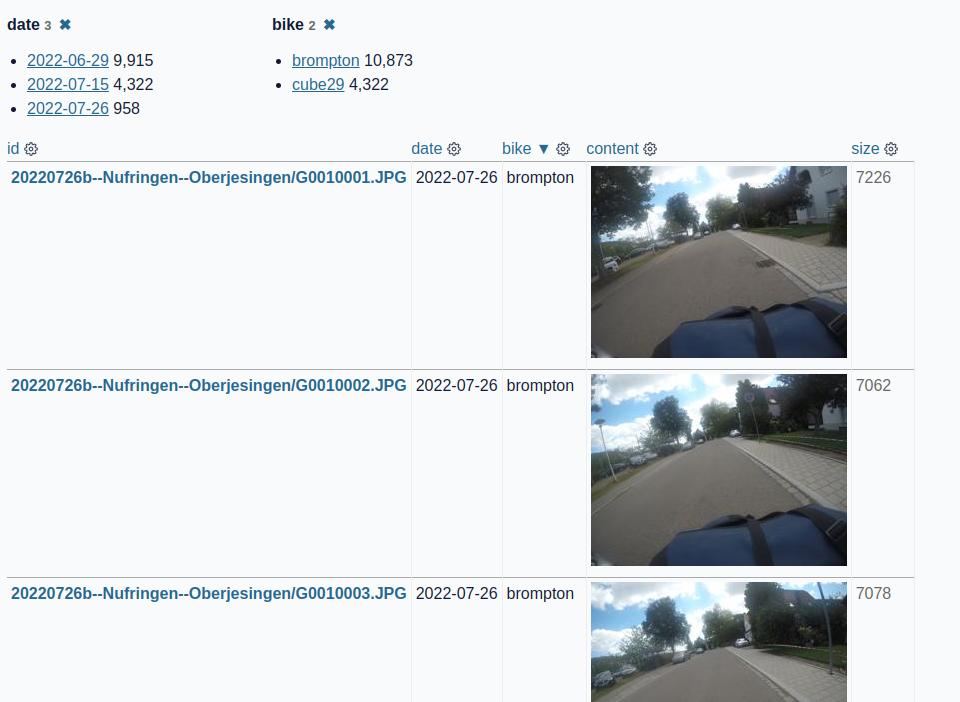